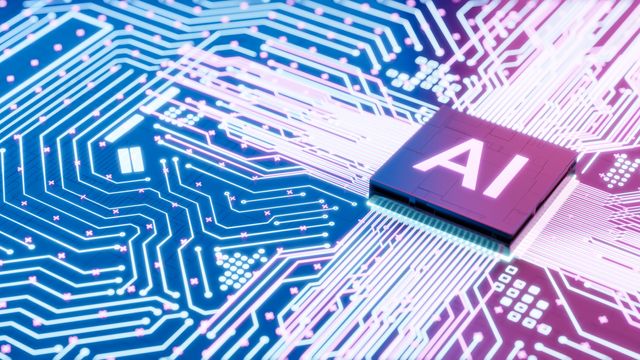
Researchers on the Department of Energy’s SLAC National Accelerator Laboratory have developed a brand new machine studying software that is ready to interpret the swinging of atomic spins in a system, enabling extra environment friendly research of complicated quantum supplies. Published in Nature Communications, the examine particulars the creation and coaching of the brand new synthetic intelligence (AI) platform utilizing “neural implicit representations,” a machine studying software that derives unknown parameters from experimental knowledge. This method has already been carried out in different scientific fields, corresponding to medical imaging and cryo-electron microscopy. The researchers say that their new software might assist to speed up the investigation of novel supplies, because it helps automate a number of the extra labor-intensive experimental knowledge evaluation inside supplies analysis. An various method to AI helpTo perceive the principles governing uncommon methods, corresponding to magnetic supplies, researchers typically wish to look at their collective excitations. In easy phrases, collective excitations are phenomena that come up at very small scales, through which a stable behaves extra like a set of weakly interacting particles in a vacuum. One instance of this is perhaps tiny adjustments within the sample of atomic spins in a fabric, which may have an effect on its magnetic properties. Understanding such properties is essential for the event of novel applied sciences, corresponding to superior spintronics units which have the potential to revolutionize how we ship and retailer knowledge. Current strategies for finding out collective excitations are extremely intricate and infrequently require a major funding of assets. Excitation spectra are usually obtained utilizing inelastic neutron or X-ray scattering methods. These are analyzed by evaluating experimental outcomes towards calculated predictions, however a paucity of accessible neutron sources has many reconsidering how such experiments could be made extra environment friendly. To scale back the intensive wait instances for quantum supplies evaluation at neutron scattering amenities, there’s a actual urge for food for brand new methods for real-time modeling and evaluation of experimental spectral knowledge.Want extra breaking information?Subscribe to Technology Networks’ each day publication, delivering breaking science information straight to your inbox on daily basis.Subscribe for FREESome headway has been made with the appliance of standard machine studying algorithms in dashing up the gathering and processing of neutron scattering knowledge. In a bid to enhance upon these efforts, the researchers from the SLAC National Accelerator Laboratory got down to assemble the same system that makes use of neural implicit representations, somewhat than conventional studying algorithms.Neural implicit representations “see” inputs as being coordinates on a map. For imaging purposes, the place a conventional image-based studying system would retailer a picture immediately, neural implicit representations create a recipe for learn how to interpret the picture by connecting a pixel’s coordinate to its coloration. With sufficient of those knowledge factors, such a mannequin can be taught to make very detailed predictions, even between pixels in a picture. “Our motivation was to know the underlying physics of the pattern we have been finding out. While neutron scattering can present invaluable insights, it requires sifting by means of large knowledge units, of which solely a fraction is pertinent,” stated co-author Alexander Petsch, a postdoctoral analysis affiliate at SLAC’s Linac Coherent Light Source (LCLS) and Stanford Institute for Materials and Energy Sciences (SIMES). “By simulating hundreds of potential outcomes, we constructed a machine studying mannequin educated to discern nuanced variations in knowledge curves which might be just about indistinguishable to the human eye,” he stated.Predictive algorithm can help with evaluationThe analysis workforce needed to design an AI software that might interpret the measurements made by the workforce on the LCLS, and recuperate the microscopic particulars of the fabric in close to real-time because it was measured. After hundreds of simulations masking a spread of various parameters that might usually be used to review quantum supplies, the researchers fed this spectral knowledge right into a machine studying algorithm. This step was performed in order that the workforce might predict solutions from concept as quickly as they measured actual spectra. For the actual spectra element, they fed in inelastic neutron scattering knowledge that had been generated by Petsch as a part of his doctoral thesis. When the workforce utilized their machine studying mannequin to the real-world knowledge, they discovered that it was in a position to efficiently overcome quite a few essential challenges with knowledge evaluation, together with background noise and lacking knowledge factors. The researchers additionally demonstrated how their method could possibly be used to constantly analyze knowledge in actual time – highlighting the potential of such a method to streamline present supplies evaluation approaches.”Our machine studying mannequin, educated earlier than the experiment even begins, can quickly information the experimental course of,” stated SLAC lead scientist Josh Turner, who oversaw the analysis. “It might change the best way experiments are carried out at amenities like LCLS.”Crucially, the researchers say that this machine studying mannequin design isn’t just restricted to inelastic neutron scattering experiments. This approach might additionally assist bypass the necessity for complicated peak-fitting algorithms or user-intensive post-processing in different scattering measurements. “Machine studying and synthetic intelligence are influencing many alternative areas of science,” stated co-author Sathya Chitturi, a PhD scholar at Stanford University. “Applying new cutting-edge machine studying strategies to physics analysis can allow us to make quicker developments and streamline experiments. It’s thrilling to contemplate what we are able to deal with subsequent based mostly on these foundations. It opens up many new potential avenues of analysis.”Reference: Chitturi SR, Ji Z, Petsch AN, et al. Capturing dynamical correlations utilizing implicit neural representations. Nat Commun. 2023;14(1):5852. doi: 10.1038/s41467-023-41378-4This article is a rework of a press launch issued by the SLAC National Accelerator Laboratory. Material has been edited for size and content material.
https://www.technologynetworks.com/applied-sciences/news/new-ai-tool-can-help-researchers-understand-quantum-materials-379882